
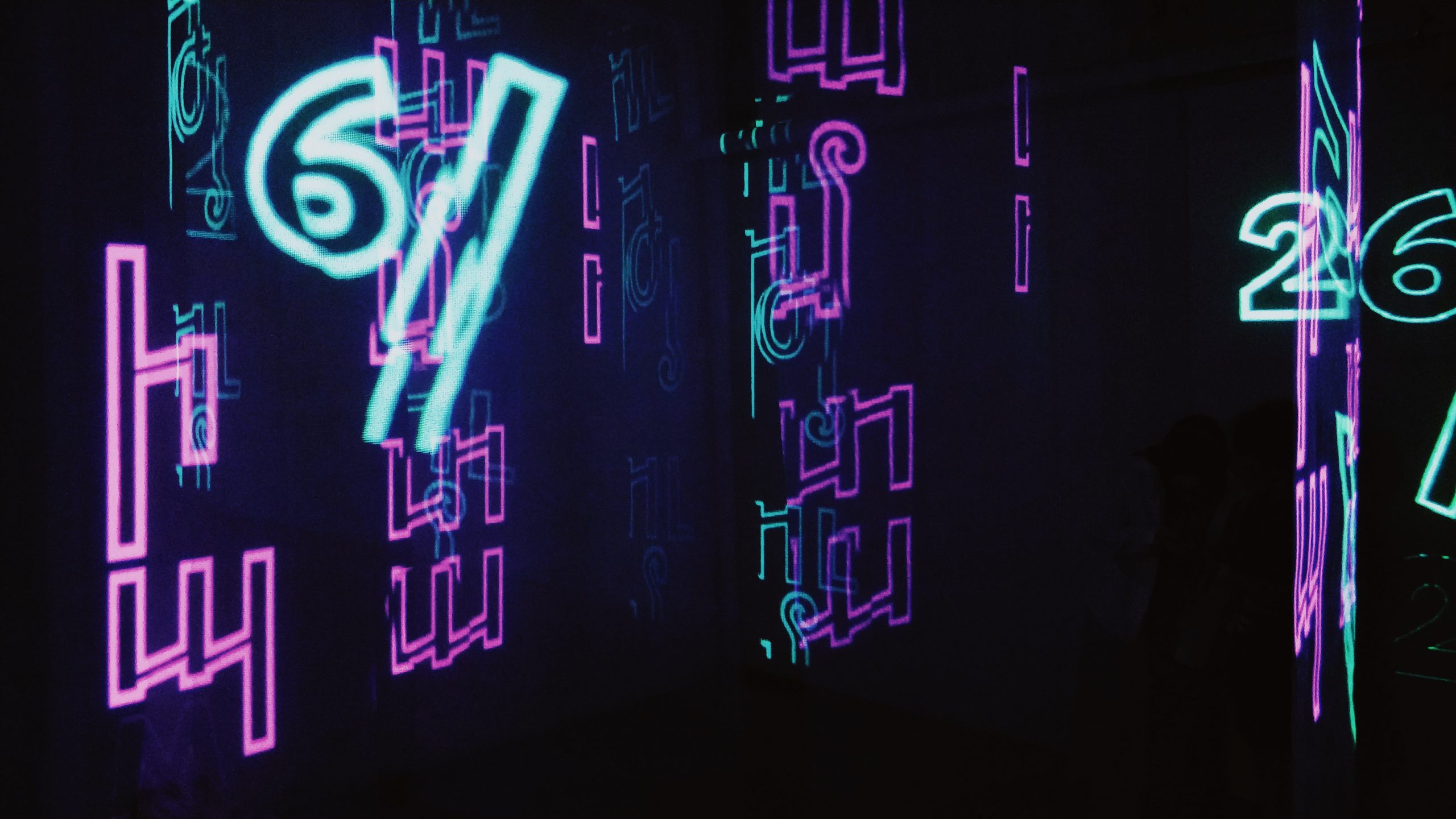
Artif Intell Mach Learn Multi Domain Oper Appl. You S, Kuo C-CJ (2019) Defending against adversarial attacks in deep neural networks. Sayghe A et al (2020) Survey of machine learning methods for detecting false data injection attacks in power systems.
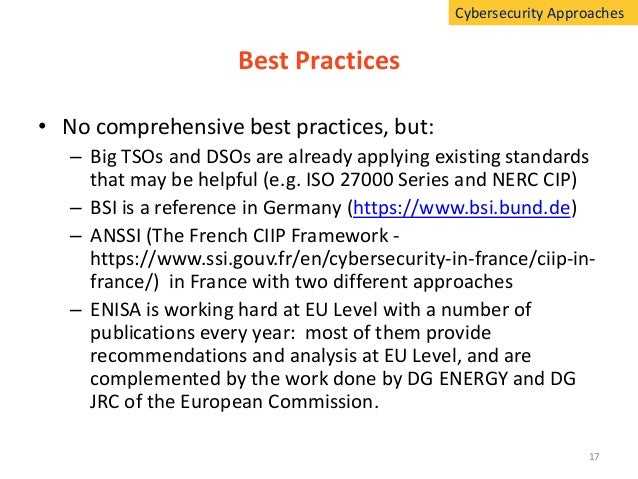
Sakhnini J (2020) Security of smart cyber-physical grids : a deep learning approach In: 2019 IEEE 7th International Conference on Smart Energy Grid Engineering (SEGE), pp 108–112, doi. Sakhnini J, Karimipour H, Dehghantanha A (2019) Smart grid cyber attacks detection using supervised learning and heuristic feature selection. Kumar A, Saxena N, Choi BJ (2021) Machine learning algorithm for detection of false data injection attack in power system. Intruders L, Grids S, Rouzbahani HM, Karimipour, Lei L (2022) Multi-layer defense algorithm against deep reinforcement multi-layer defense algorithm against deep reinforcement learning-based intruders in smart grids. Saghezchi FB, Mantas G, Violas MA, de Oliveira Duarte AM, Rodriguez J (2022) Machine Learning for DDoS Attack Detection in Industry 40 CPPSs. Qi R, Rasband C, Zheng J, Longoria R (2021) Detecting cyber attacks in smart grids using semi-supervised anomaly detection and deep representation learning. In: 2019 2nd International Conference on Power and Embedded Drive Control (ICPEDC), 5(2020): 546–551. Rajendran G, Sathyabalu HV, Sachi M, Devarajan V (2019) Cyber security in smart grid: challenges and solutions. When supervised learning and hybrid methods are utilized in a simulation exercise, the performance of the classification techniques that are used to detect FDI attacks is significantly improved.Įl Mrabet Z, Kaabouch N, El Ghazi H, El Ghazi H (2018) Cyber-security in smart grid: survey and challenges. The accuracy of each detection method in terms of its classification is what is used to evaluate them. Using data obtained from the smart grid, an analysis of the adaptability of these approaches is carried out. These strategies are based on SVM-boosting algorithmic frameworks. In this paper, six alternative supervised learning (SVM-FS) hybrid methods, each with its own set of six unique boosted and feature selection (FS) procedures, are analyzed. The detection of FDI assaults can be accomplished using a multitude of methods, one of which is machine learning. The faulty data detection methods that are now in use are unable to detect covert FDI attacks, making it impossible to do so (El Mrabet et al. False data injection, sometimes known as FDI, is a common form of assault that is launched against smart grids.
